Modern Data Platform: Characteristics, Whats, and Whys of the Modern Data Platform
This is the first article in the series on building modern data platform.
If you Google “modern data platform” you will get a lot of advertisement and, ironically, a promotion of a book “Hadoop at Scale”.
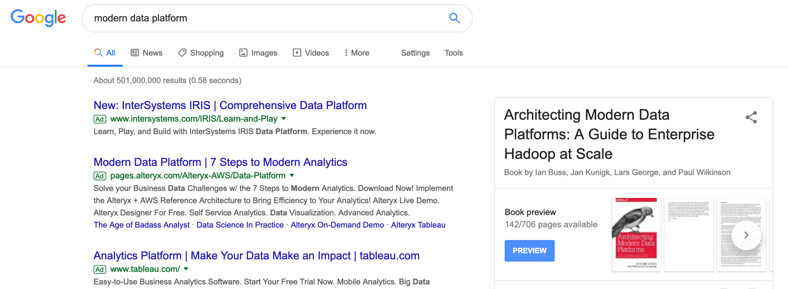
Hmm, Google seems confused. Let’s try to agree on what modern data platform is. In 2017 Planning Guide for Data and Analytics, Gartner found that “Data and analytics are at the center of every competitive business.” In 2019, US Navy implemented consolidated data platform to enable self-service analytics. It’s hard to find organization today that is not aspired to be data driven. One of the characteristics of the modern data platform is focusing on solving this challenge by enabling data at scale – scale of the audience and scale of the data.
Scale of audience means various use cases, personas, and usage patterns. Data engineers, for example, focus on writing code for complex batch ETL processes. Data analysts need to query structured data from various sources and receive results with a speed of thought. Data scientists need an ability to experiment with various machine learning frameworks and run training processes against a wide variety of mostly unstructured data.
Scale of data is well covered by the famous Three Vs – Volume, Variety, and Velocity. The plot below is from a 2012 article by Diya Soubra. The Three Vs is a very important characteristic of not only data, but also of the modern data platform.
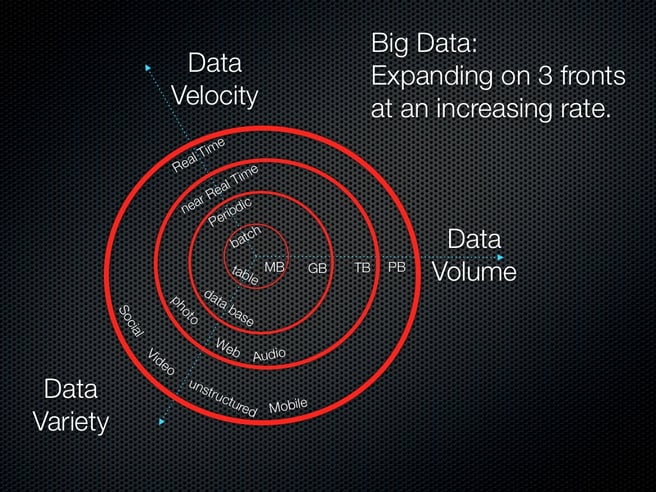
With exponential growth of data, making data discoverable becomes another important characteristic of the modern data platform. Various user groups need to not only discover data but also properly and consistently interpret the data. The same data may have different meaning for different user groups. Making the data catalog somewhat decentralized and crowd-sourced within your organization will allow for multi-dimensional while still consistent interpretation of the data.
The modern data platform must also provide data federation and data virtualization capabilities to allow users to easily analyze data with a single tool. Without these capabilities, users would need to know where the data is located, the data format, and what tools need to be used to access data from each source; data consumers would need to copy data from multiple sources into a single place or depend on data engineers to create and execute ETL processes. Data federation and virtualization help to solve these problems.
It’s not an exaggeration that the total cost of ownership (TCO) is one of the most important characteristics of the modern data platform. The operational part of TCO is a combination of the ongoing cost of the environment and the cost of labor. With extreme variations of the workload, modern SLA will drive the fixed cost very high in a traditional environment. Elasticity allows to drastically reduce that cost by transitioning it to variable cost and must be a core principle of the modern data platform. Self-service is a principle that drives tool selection and helps with significant increase of productivity and reduction of both fixed and variable costs. Following these two principles will help to increase data platform adoption and reduce TCO at the same time.
While a modern data platform serves to democratize data, it must also be secure and support users and data in various geographical and legislative regions through data governance.
To summarize, the modern data platform must:
- enable data across your entire organization,
- allow to discover data easily,
- be self-service and scalable,
- support extreme variations in the workload,
- be cost and resource efficient,
- cover data warehouse and data lake use cases,
- allow to federate your databases, data warehouses and data lakes and use them in a single query independently on whether the data is located on-prem or spread across multiple clouds,
- be secure and still support users and data in various geographical and legislative regions,
- enable predictive analytics and other data science use cases,
- utilize modern tools and take advantage of the cloud offerings.
Now, I want to shift gears a bit and talk about execution. While an alignment on the strategic business objectives is fundamental for any project, it’s often missed. I witnessed a large IT organization failing two-year initiative of modernizing their data platform. The program was funded by IT and their main objective was to reduce the cost through migrating data warehouse to data lake. However, IT management tried to sell to the business that their objective was meeting business demand on increasing data volume, velocity and variety. When the project was delivered to the business, the misalignment of objectives became apparent and the project failed to deliver up to the expectations of the business. The funding was stopped, people were let go. It was a great lesson on criticality of the alignment on the strategic business objectives for building a modern data platform.
Developing measurable and realistic metrics reflective of the desirable outcome is imperative for the ability to report the status of the initiative and recognize successful implementation. Without properly defining metrics, the initiative might be successfully achieving the objective and produce desirable outcome and still might be seen as failing if measured on unrelated or unrealistic metrics. It’s also possible that the initiative will be driven towards achieving poorly defined metrics and not achieving desirable outcome.
Building a modern data platform is a long-term initiative. Requirements and the ecosystem will be a moving target. It is important to have a roadmap which will reflect a visionary direction and define milestones for small but important wins along the way. It’s a good practice to do a reality check and review the roadmap regularly. It will help the initiative to stay on top of moving targets, reset expectations, and mitigate risks.
The selection of tools today is the largest it has ever been, and the market space is very dynamic with new tools popping up almost every day. Most of these tools are open source and free. Choosing the right tools for the job is vital. However, it’s not efficient to implement open source tools in their raw form. There is a number of vendors offering these tools in a variety of ways. Choosing the right vendors is as vital as choosing the right tools.
To draw the bottom line, building a modern data platform is a large, complex, expensive, and risky undertaking. It’s not surprising that most of these initiatives are struggling to say the least. According to Gartner survey, only 15% of big data implementations ever reach production. Forbes published a research with a bit rosier picture, but still most metrics fell below 30%.
I hope this article is informative. Whether your experience correlates with mine or not, please share your thoughts. The next article is about the Cloud as pertained to Modern Data Platform. Stay tuned!